Data is omnipresent and companies are waking up to its potential. 5 exabytes of data is produced every day, and 463 exabytes of data will be generated daily by people across social media, communication and video sharing by 2025. Still, many companies struggle to effectively measure and report on the business value of their investment in data and its analysis.
Organizations are drowning in data but starving for insights
According to the Harward Business Review study, while 80% of companies agree that it is critical to extract value from data, only a quarter of the companies claim to be able to do so. They have the necessary tools and capabilities needed for data-driven innovation but fail to define a clear data strategy that will help them translate the information into business value.
Companies find it difficult to clearly determine the specific problems they are solving with data before diving deeper in product design and development. They get stuck in not having a clear view of how data engineering could improve their business. Consequently, they end up building architecture that is not flexible. By the time they realize the immense value data can bring to their business, it might be too late, and they might need to start the entire project from scratch, powered by the knowledge and skills of experienced data engineers.
On top of this, until recently, the critical role of data engineers was hidden under the vail of ever-accelerating push of data scientists. According to Gartner Data Team Management Survey, fewer than half of respondents invest in this role. With data now being everywhere, companies are increasingly realizing that it does not bring any value if not leveraged to make certain actions and build infrastructures that would then lead to more efficient outcomes and better decision making.
Paving the way from data insights to operation
If organizations want to realize the full potential of data engineering, they need to break the boundaries of possible and explore beyond what’s visible on the surface. They need to dive deeper, challenge the status quo, and ask questions of their data capabilities and how it can help unlock higher value through new game-changing use cases.
We identified three ways how businesses can challenge their “best practices” and transform their current processes to capitalize on the rise of data-driven enterprise. We talked with our colleagues-engineers, who shared these tips based on their experience working on some of the most thought-provoking projects where data engineering played a major role.
1. Identify a specific challenge you want to solve, and consider data engineering from day one
Oliver Kosta Zivic, Data Engineering Tech Lead at HTEC, says that to capture data’s full potential, businesses not only need to undergo a certain degree of technological and organizational change, but they also have to show eagerness to go where data takes them.
“If business leaders want to realize the full potential of their data, they need to be aware of the importance of handling data properly from the start. No matter what a company decides to scale up, they will always have to face the challenge of managing large amounts of data at some point. Unless they take care of their data from the beginning, they will have to face growing pains.”
So, I would even go so far as to say that we should all have T-shirts with a caption “Consider hiring a data engineer because you will need them. You just don’t know it yet” printed on it. Jokes aside, at its core, it all comes down to raising awareness that organizations essentially need data engineers from day one. Otherwise, they risk getting swamped by the sea of data which then takes them back many steps behind, increases the costs, and leads to undesired results.”
Business processes often keep changing all the time, thus opening doors to new opportunities and data points that companies might not consider from the very beginning. “Data engineers can often find correlations in data that point to certain conclusions that might have a massive impact on the client’s business. This might accelerate innovation and change the process, or even the entire client’s business model. The things that used to take days to complete, now take only a few minutes. Led by our experience, to reach success, companies need to focus on a specific and real business problem they want to solve to be able to create a new stream of ROI.”, says Oliver.
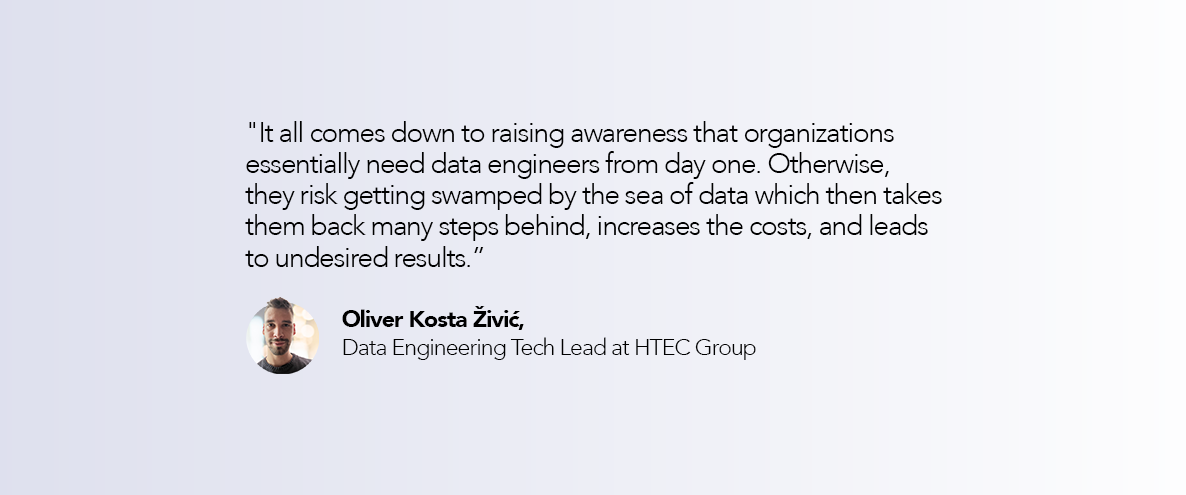
2. Data-Engineering as a Way to Push AI Forward
Data is everything in modern-day machine learning. However, it is often neglected and not handled properly in AI projects. As a result, we spend hours and hours tuning a model built on low-quality data. This is why the accuracy of your model is significantly lower than expected — it has nothing to do with model architecture or parameter tuning.
Aniko Kovac, ML Engineer turned PM for data teams at HTEC Group, points out that data engineering is a way to push AI forward.
“The answer to pushing AI forward now and over the coming years can be found in a data-centric approach. This is a global trend driven by Dr Andrew Ng. Andrew presented an interesting topic, From Model-Centric to Data-Centric AI, where he explains how important data is for machine learning (ML), even much more important than the ML model itself. Essentially, while model-centric AI asks how you can change the model to improve performance, data-centric approach asks how you can change or improve your data to improve performance. Andrew proposed that data-driven AI is the future. For instance, he inspected steel sheets for defects where the baseline system had 76.2 % accuracy and the goal was to reach 90% of accuracy. He tried to do a model-centric improvement (training new models, new architectures) and he got zero improvement in accuracy. On the other hand, when he tried a data-centric approach, he got 16.9 % improvement, which caused the overall system to be over 90% accurate – pretty high result for the industry.”
Take a look at the following image, taken from the mentioned session:
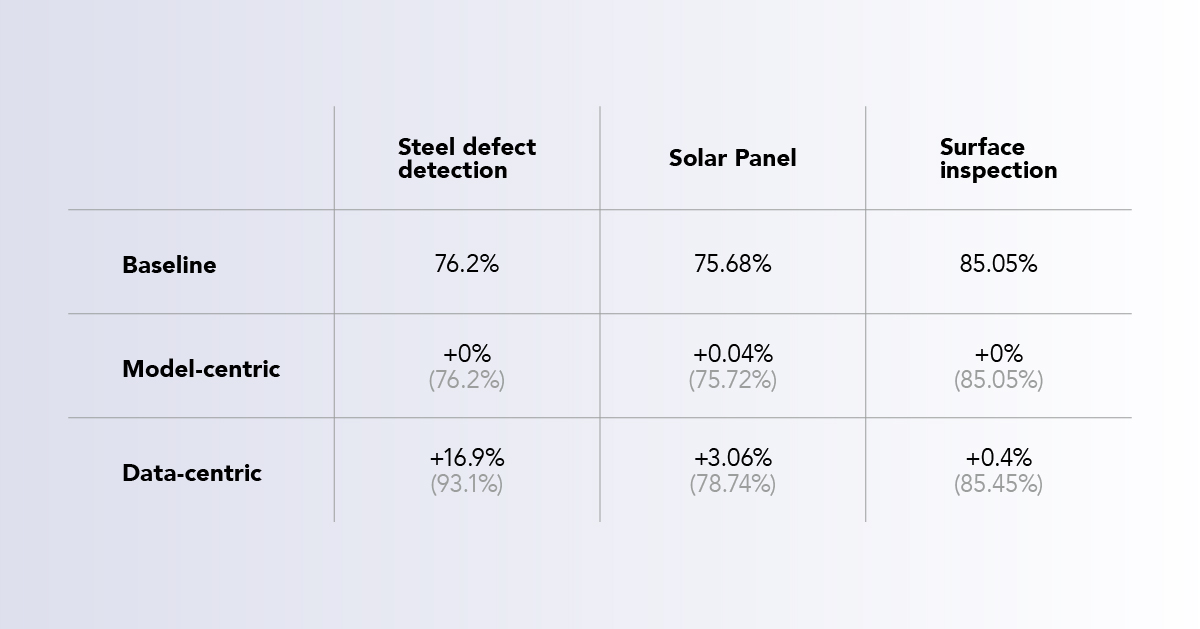
Aniko explains that data engineering will play a significant role in processing data that can help with data-centric AI.
“With data-centric AI development, teams spend much more time on ingesting, pre-processing, augmenting, managing, and monitoring data, because data quality and quantity are becoming crucial for successful results. This can help companies improve their processes and automatize everything from factories to streaming platforms. The advantages of becoming more data-centric are numerous, ranging from improved reporting speed and accuracy to better-informed decision-making.”
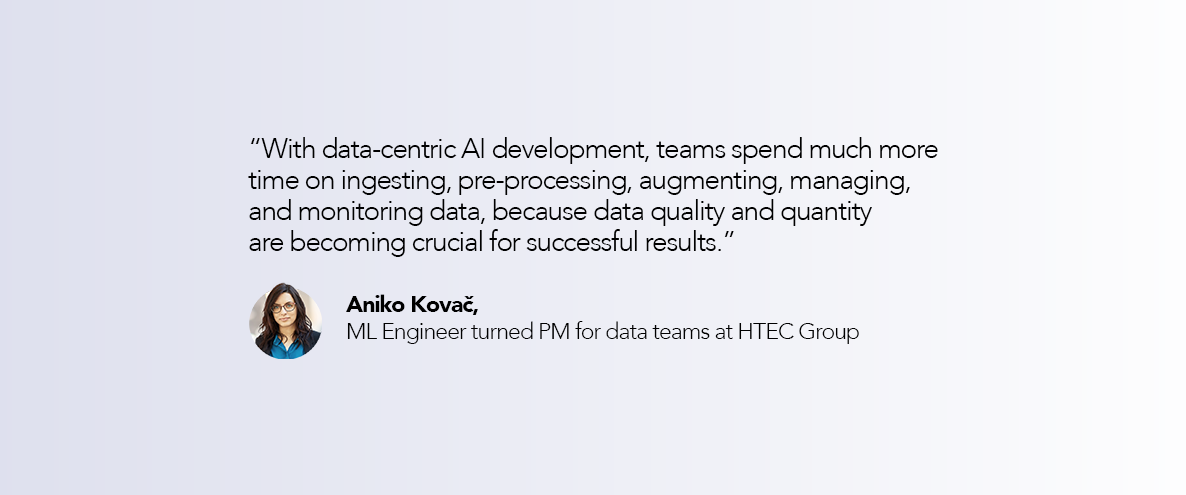
3. Get more value out of your data in the cloud
When an organization leverages data strategically, this helps them strengthen customer satisfaction and drive competitive advantage. But what road should companies take to get there?
Dragan Beric, Data Engineer and Delivery and Tech Lead at HTEC, points out that Cloud technology is essential to manage data effectively at scale.
“Data storages evolution gave us the opportunity to collect data coming from any device in the world and store it before leveraging it to create business value. That schema-on-read model really increased the volume of data in the world that is currently stored. Companies are switching more to this model as they do not want to miss any event/transaction/information that their devices produce. By creating a centralized data storage in the Cloud, different personas including data scientists and data analysts within the enterprise company can have access to different data pipelines based on their needs. For instance, a business analyst would like to view data in the graphs or trends to be able to track KPIs, and a data scientist would like to use data to create and train their machine learning models. At its core, data engineering can provide a good support for different data driven solutions by creating a well-organized Cloud storage and implementing data pipelines.”
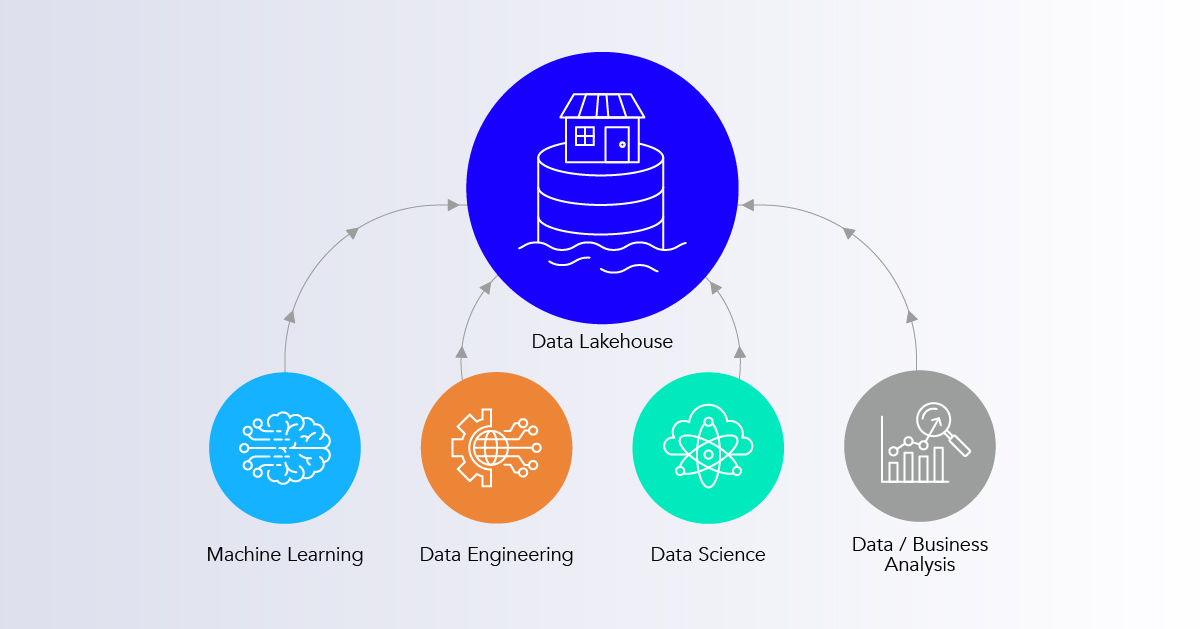
A project we undertook with our long-lasting client Marlink demonstrates the power of Cloud technology for managing huge amount of data.
“Top challenges for Enterprise data usage are: Access (Can I access this data with my favourite data tool?), Reliability (Is my data correct?) and Timeliness (Is data fresh?). As Marlink has several systems that create data, we faced the same challenges as we really wanted to democratize the data and make it available for every persona in the organization. Switching from 2 tier architecture to the Lakehouse architecture, leveraging the best from Data Lake & Datawarehouse, we created a centralized storage with all the data at one place in the Cloud. That single source of truth ensured that all processes and personas that are using the data work with fresh, cleansed and correct data, with minimum effort for accessing the different system and type of data. Now we have a data platform that can serve different data engineering and integration processes, data analysis pipelines or machine learning models.”
With an organization’s data in the cloud, it is readily available to those who can best use it to drive greater value. This helps organizations build a more responsive supply chain and improve interactions with their customers. Companies should consider ways how to go faster in this fast-paced digital ecosystem. Building an effective data strategy that uses Cloud as a solution is the key.
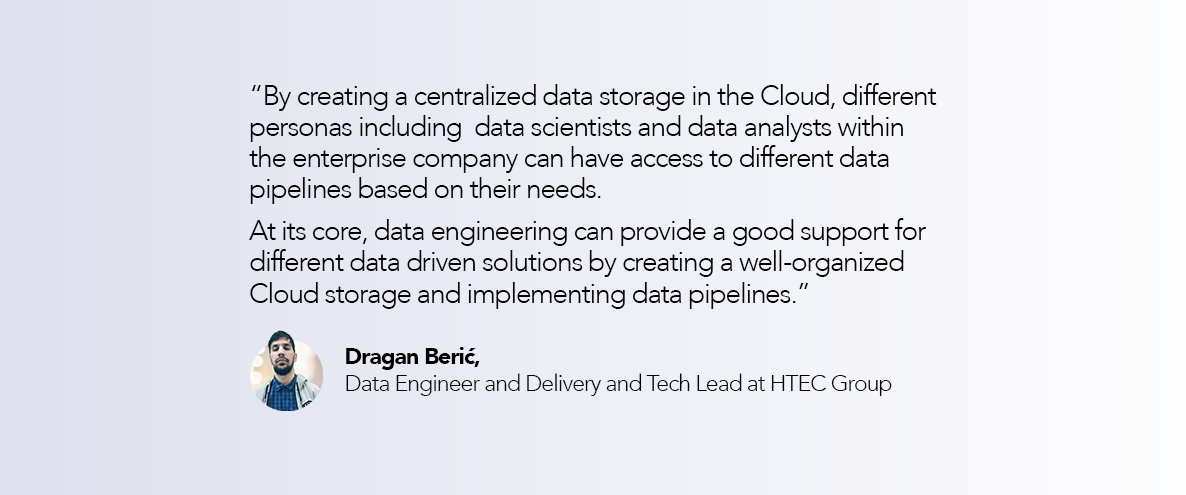
Let’s put data to work
Businesses are usually learning the hard way that data means nothing if actions are not taken based on it. Great data science models and insights only bring value if they reach the end-user. Don’t let your data reside in notebooks. Set up teams together with data scientists, dana analysts and data engineers to come up with the efficient data strategy that will pave the way from data insights to operations. HTEC Group can help you on this journey by setting up processes and infrastructure that will move data science models smoothly and securely from development to production.
Talk to us to learn how we can help you put data to work.