Welcome to the dawn of a new era in healthcare, where cutting-edge technologies and innovative approaches are transforming the landscape of the medical device industry. In this article, we delve into the most groundbreaking trends shaping the future of healthcare, from the integration of artificial intelligence and machine learning to the rise of telemedicine and remote monitoring. These remarkable advancements are revolutionizing patient care, enhancing diagnostic accuracy, and unlocking the potential of personalized medicine.
Driving the next wave of digital innovation in healthcare
As we traverse this disruptive moment in healthcare, it’s critical to understand the driving dynamics behind the medical device industry’s booming demand for machine learning (ML), deep learning (DL), and artificial intelligence (AI). A number of variables, ranging from clinicians, providers and payers to healthcare professionals, are driving demand for innovative technology that gives new capabilities and insights.
The key motivator for physicians is the desire to improve patient outcomes and provide more efficient, cost-effective treatment. By leveraging the power of machine learning and artificial intelligence, providers may speed diagnostic processes, spot trends and anomalies more rapidly, and make data-driven decisions that lead to better treatment plans and overall care. Machine learning and Artificial Intelligence are poised to transform the healthcare landscape, but rather than replacing clinicians, they will serve as valuable tools to enhance their capabilities. It is important to recognize that clinicians possess a unique understanding of the patient’s context, including their medical and family history, which allows them to provide a more personalized approach to care.
On top of this, healthcare workers, such as physicians, nurses, and specialists, are driven by the desire to use cutting-edge technology to provide the best possible treatment to their patients. Machine learning, Deep learning and Artificial intelligence can supplement their knowledge by providing useful insights and clinical decision support, allowing them to make more accurate diagnoses and enhance treatment plans. By offering rapid and potentially more accurate analysis of complex medical data, AI can help healthcare professionals make better-informed decisions, ultimately leading to improved patient outcomes.
Payers, on the other hand, are seeking strategies to improve resource allocation, decrease fraud, and reduce medical claim expenses. AI-driven analytics and automation can assist in identifying patterns of misuse, flagging potential errors, and enabling more accurate risk classification, resulting in a more efficient healthcare system.
To summarize, the growing market for ML and AI in the medical device business is being driven by a common goal among providers, payers, and healthcare professionals to transform the healthcare landscape. By embracing these game-changing innovations, stakeholders across the healthcare spectrum can collaborate to build a more efficient, effective, and patient-centered system that raises the bar for all.
The market for ML and AI is depicted in the figure that can be seen below. [2]
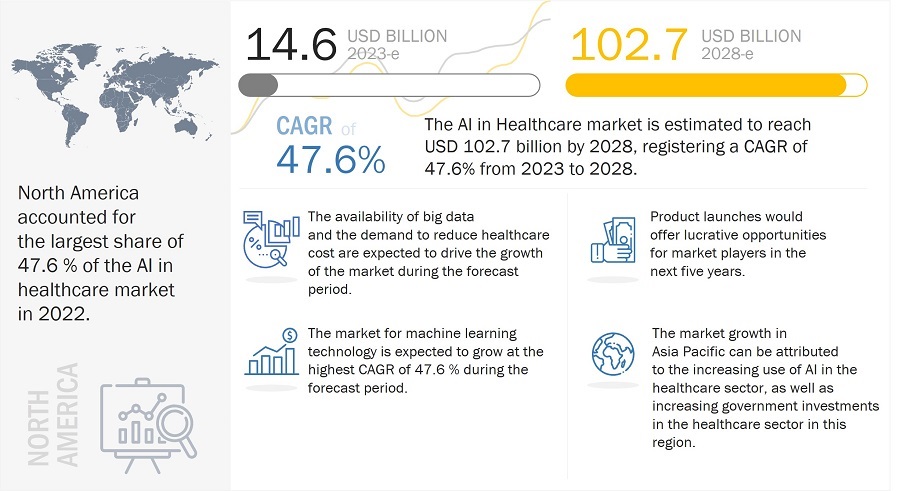
Figure 2: AI in Healthcare Forecast (1), Source — Markets and Markets
By offering rapid and potentially more accurate analysis of complex medical data, AI can help healthcare professionals make better-informed decisions, ultimately leading to improved patient outcomes. Currently, AI models face limitations due to the prevalence of unstructured and poor-quality data, which makes it challenging to fully train them with a deep understanding of the patient context.
As we acknowledge the current constraints of AI, we can appreciate the complementary relationship between AI-driven technologies and the indispensable expertise of clinicians in providing comprehensive patient care.
Join us as we embark on this inspiring journey to explore the top trends driving change and challenges in the medical device industry and witness the unfolding of a new chapter in human health and well-being.
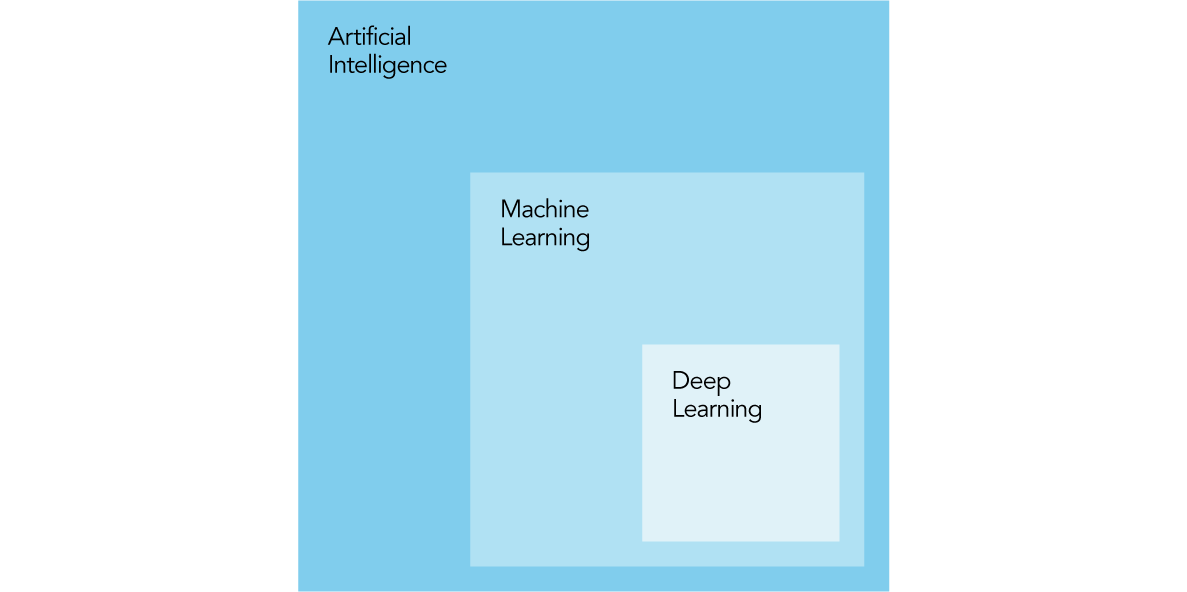
The role of machine learning and Artificial Intelligence in diagnostics and therapeutics
Far from being yet another false promise, we are currently witnessing a genuine revolution as ML and AI technologies rapidly evolve and mature.
A critical factor driving innovation is the availability of high-quality, structured data without neglecting unstructured data [1]. The success of these breakthroughs relies heavily on access to vast amounts of accurate and well-organized data, which enables AI algorithms to learn, adapt, and improve. Consequently, organizations and researchers with the privilege of accessing such valuable data resources will be at the forefront of this transformation, leading the change.
With breakthroughs emerging monthly, it’s becoming increasingly challenging for established medical device manufacturers and regulators to keep up with the pace of innovation. As we continue to investigate the developing story of AI and ML in healthcare, it’s critical to recognize that projecting the precise trajectory of these technologies and their impact on the medical device sector is a difficult task. The rapid pace of technical innovation, along with ever-changing legislative frameworks, makes pinpointing particular outcomes with perfect confidence difficult.
We can, however, see probable future directions for AI and ML in diagnostics and therapy by studying existing trends and relying on insights from professionals and researchers.
In the following paragraphs, we will explore some of these fascinating possibilities, keeping in mind that the field’s dynamic nature necessitates that our understanding and expectations remain fluid and adaptive.
Improved diagnostic accuracy, speed, and efficiency
Medical diagnostics involves examining a medical history and patient’s symptoms, and testing results to identify the root cause of a health issue and recommend appropriate treatment. Procedures like imaging diagnostics (X-rays, MRI, CT), blood tests, and biopsies all play a part in this process.
There have been countless studies done about the impact of AI and ML in medical diagnostics. A systematic literature review sponsored by Sejong University [3] found out that AI plays an important role (65% of cases) in future medical diagnostics.
AI algorithms can analyze medical images, patient data, and vital signs to support clinicians in making more informed decisions about patient care. By incorporating multiple data sources, healthcare providers can better understand patients’ health and the origins of their symptoms. This approach can improve diagnostic accuracy and reduce misdiagnoses.
Emerging AI technologies, such as quantum artificial intelligence (QAI), hold great promise for accelerating data processing and improving diagnostic outcomes.
Research has shown that AI systems can achieve diagnostic accuracy comparable to or even surpassing the results achieved by human experts in some cases, leading to faster diagnosis and better patient outcomes.
This systematic review and meta-analysis compare the performance of deep learning models against healthcare professionals in detecting diseases from medical imaging. The study found that deep learning models achieved comparable accuracy with healthcare professionals across various medical imaging tasks [4].
Another study researching the use of AI for colorectal carcinoma diagnosis found that quantitative pathologic analysis of colorectal carcinoma images can provide a wealth of information that can be used to augment routine pathologic reporting and that a prognostic model using AI improves prediction of recurrence-free survival. [5]
Before moving on to the next topic, let me go back to the Sejoung University study. As part of their conclusion, they discussed on why we need AI in medical diagnostics, and what is its impact:”
The best part of implementing AI in healthcare is that it helps improve various areas, including illness detection, disease classification, decision-making processes, giving optimal treatment choices, and ultimately, helping people live longer. In terms of disease diagnosis, AI has been used to enhance medical diagnosis (Chen et al. 2019a, b).” [3]
Predictive analytics
AI can leverage vast amounts of data from Electronic Health Records (EHRs), medical literature, and other sources to identify patterns and trends that can help predict disease onset, progression, and response to treatment. This can enable healthcare providers to make more informed decisions about patient care, potentially improving outcomes and reducing healthcare costs.
As OpenAI grows and develops, the future of AI-based medical diagnostics is likely to be characterized by continued growth and improvement. In research, quantum AI is being utilized to expedite training and produce rapid diagnosis models as quantum computers can process massive volumes of medical data in real time, allowing for more accurate and efficient diagnosis.
DeepQA from OpenAI and DeepMind from Google both use general AI. General AI for medical diagnostics enhances accuracy, speed, and efficiency while providing healthcare personnel with important insights and assistance in patient diagnosis and treatment. By analyzing huge amounts of medical data and discovering patterns and links, general AI for medical diagnostics might improve patient outcomes and healthcare efficiency. To develop tailored treatment procedures, AI-based technologies might assess a patient’s medical history, genetics, and other factors and bring the future of genomics into focus [6].
Precision medicine
AI can analyze genetic, molecular, and clinical data to identify the most effective treatments for individual patients, considering their unique genetic makeup and medical history.
In a general sense, it is anticipated that AI will assist in the realization of the promise of precision medicine in three primary areas:
- the prevention of disease
- individualized diagnosis
- personalized treatment
Predictive models rely on historical patient data to uncover trends that predict future results. Machine learning integrates ongoing patient data to improve individual forecasts. Predictive models assist doctors in understanding the risks and probable courses of illness development and healthcare requirements, allowing them to build more effective treatment regimens.
A modern type of information known as recreational data, enabled through IoT, will become increasingly significant in the healthcare profession. The Internet of Things (IoT) is a growing network of sensors and technologies that collect data and are used in our daily lives. Wearables and smart systems are two common examples of gadgets that provide continuous data streams that can be used to gain a better understanding of our way of life. These datasets, together with standard healthcare datasets, have been utilized to gain a better understanding of the COVID-19 pandemic and act as disease prevention tools. This is just one of the most recent examples of AI usage for prevention.
Furthermore, Diagnostic AI models evaluate symptoms to help clinicians correctly diagnose health issues. Diagnostic analytics are typically utilized to diagnose individuals who already have serious symptoms. However, AI allows diagnostics to play a bigger role in preventative medicine.
Screening (preventive diagnostic model innovations can detect dangerous illnesses in their early stages and, in certain circumstances, earlier and more correctly than traditional health checks. A study focused on individualized diagnostics for detecting lung cancer found that machine learning has a tremendous impact on early detection [8].
It is now possible to receive individualized medical care that is informed by AI-based models, and this trend is not going away anytime soon. According to the findings of a study that focused on individuals with dementia, treatment plans that were personalized using AI performed far better than those that were created in the conventional manner.[9].
Drug discovery and development
Preclinical drug discovery takes around five and a half years and costs about 30% of the total cost of pharmaceutical development [31]. Efficacy, safety, and developability are all improved repeatedly and sequentially. Millions of molecules are investigated, hundreds are created, and the vast majority fail in preclinical or clinical trials. The success rate of R&D-to-clinic translation is less than 10%, and the main causes of attrition are safety risks and low efficacy.
Everybody is looking for drug research to produce novel strategies for increasing pharmaceutical development success, lowering medicine prices, and accelerating drug delivery. Because failures account for so much of the expense of research, methods that increase our capacity to forecast which compounds will succeed early on can have a disproportionate influence on increasing the yield of new medications. To better meet patients’ urgent needs, researchers must go beyond the traditional slow, sequential, and expensive drug development paradigm, which costs billions to build a new molecular entity due to failures.
AI can accelerate the process of drug discovery by analyzing large datasets to identify potential drug candidates and predict their efficacy, toxicity, and potential side effects. This can help reduce the time and cost associated with bringing new drugs to market and facilitate the development of more targeted therapies [10].
Morgan Stanley predicts that AI in the pharmaceutical industry will be a $50 billion market [11].
Virtual health assistants and chatbots
AI-driven virtual health assistants and chatbots can provide patients with immediate access to medical information and support, helping them better understand their conditions, manage their symptoms, and adhere to treatment plans. This can lead to improved patient engagement and more effective self-management of chronic conditions.
Natural Language Processing (NLP) is likely to transform virtual health assistants and chatbots by improving their ability to recognize and interpret human language. This innovation will considerably improve the quality of interactions between users and these digital tools, resulting in a more human-like and tailored experience. As NLP algorithms improve, virtual health assistants and chatbots will be able to interpret increasingly complicated medical phrases, recognize context, and participate in more sophisticated dialogues with users. Patients will be able to obtain correct information, ask questions, and receive personalized counselling on-demand as a result of increased communication. Furthermore, healthcare providers may use smart virtual assistants to automate administrative work, improve patient involvement, and concentrate on providing high-quality treatment. The use of NLP in virtual health assistants and chatbots heralds a new age of efficient, tailored, and easily available healthcare support for both patients and clinicians [12].
The application of NLP techniques in healthcare is not new; for years there have been endeavors to utilize this technology to streamline manual processes and help healthcare workers spend more time focusing on their patient’s problems rather than administration. With the newest trends and achievements in AI, specifically in LLMs, the opportunities grow. Not only will there be more sophisticated solutions for easier understanding and providing context around medical content, but the entire content understanding will get a much deeper semantic dimension. Consolidation of information flowing from different sources, causal analysis, and predictive and prescriptive modeling, will become decision accelerators and integrate deeply into day-to-day operational workflows. This advanced technology will enable clinicians to be more efficient in their job, and patients to receive the best possible care.
Engineering and Delivery Manager at HTEC Group
The growing impact of AI in medical device industry
In 2021, AI in healthcare market was worth around 11 billion U.S. dollars worldwide. It was forecast that the global healthcare AI market would be worth almost 188 billion U.S. dollars by 2030, increasing at a compound annual growth rate of 37 percent from 2022 to 2030. The graph below shows AI in healthcare market size worldwide from 2021 to 2030 (in billion U.S. dollars).
AI in healthcare market size worldwide from 2021 to 2030 (in billion U.S. dollars)
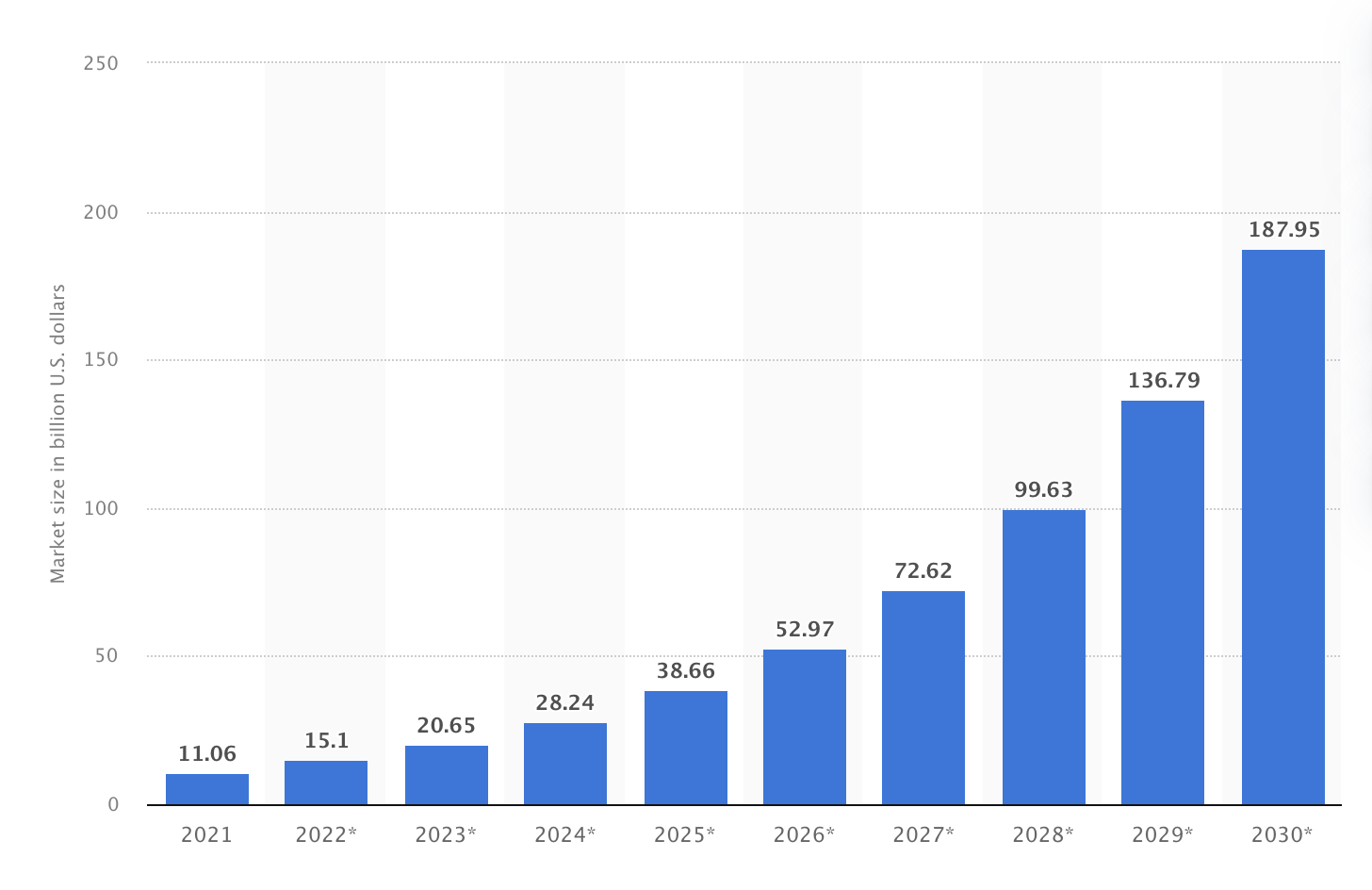
Source: Statista
Artificial intelligence (AI) is similar to the discovery of the wheel in that both have far-reaching effects across multiple industries, propelling growth and innovation. The wheel changed transportation and manufacturing, and AI is revolutionizing problem-solving, data analysis, and decision-making in a variety of industries, including healthcare and pharmaceuticals. AI has the ability to increase diagnosis accuracy, optimize clinical workflows, and enable personalized therapy in healthcare, ultimately leading to better patient outcomes. Meanwhile, AI is expediting drug discovery, optimizing clinical trials, and enabling more efficient manufacturing processes in the pharmaceutical business.
The effect of AI is growing, and as we learn more about its potential, we can expect to see even more profound discoveries and breakthroughs that will change the future of healthcare, pharmaceuticals, and countless other industries.
The impact of telemedicine and remote monitoring on medical devices
Telemedicine and remote monitoring have emerged as transformative forces in the healthcare landscape, redefining the way medical devices are designed, utilized, and connected. These innovative technologies have expanded the reach of healthcare services, allowing patients and healthcare professionals to engage in virtual consultations and monitor health conditions from anywhere at any time [13].
Remote patient monitoring (RPM) will advance telemedicine. RPM lets patients track their health using a device that sends data to their phones or tablets. Digital blood pressure cuffs, heart rate monitors, and insulin pumps are examples. RPM systems let patients communicate real-time physiologic data to their doctors to simplify monitoring. RPM progresses because of patient adoption of new technology. More than three quarters of hospitals anticipate RPM to match or surpass in-patient within five years [14]. VivaLNK found that two-thirds of 40-year-olds would wear an RPM device to reduce medical visits. New RPM technologies are constantly releasing new monitoring capabilities and telemedicine will continue this trend.
Wearable sensors and smart home-based medical equipment transmit health data to healthcare providers for continuous evaluation and timely interventions. Despite being often discarded as unreliable by physicians, studies have found that wearables have more strengths than shortcomings. In fact, a literature review study [16] found out that:
- 8.9% (16/179) of the studies, mentioned no strengths of wearables
- 55.3% (99/179) of the studies mentioned no shortcomings
Consumer-grade wearables are enhancing telemedicine and remote monitoring by offering more health insights and empowering patients to manage their health [15]. To further support the argument, the figure below lists all the strengths and shortcomings according to the afore-mentioned study.
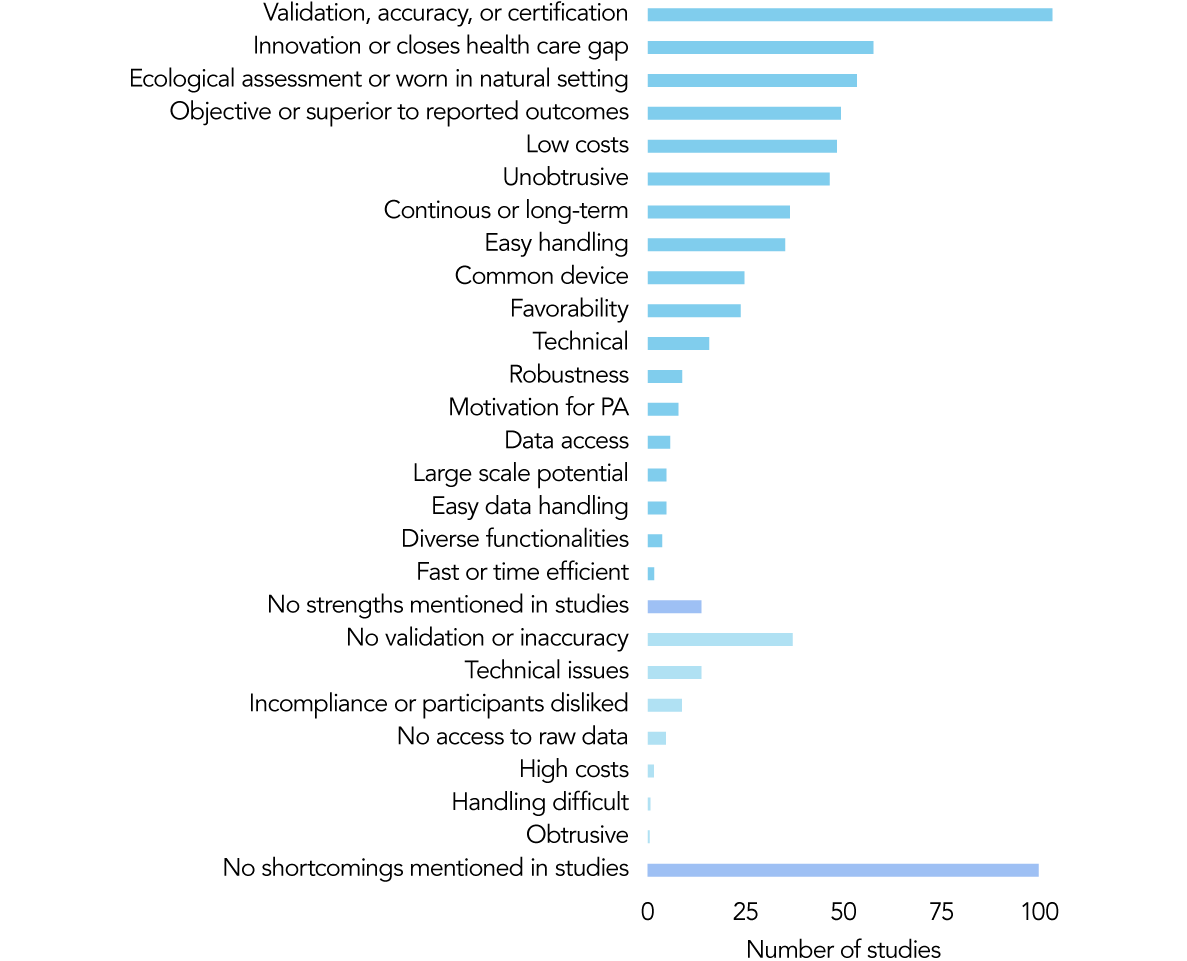
Figure 1: Chart of reported strengths and weaknesses of wearables as mentioned by authors. PA: physical activity, Source — JMIR Publications
As these wearables get more advanced, they can complement medical-grade remote monitoring devices and telemedicine platforms to capture health metrics and detect early health concerns. Integration with telemedicine and remote monitoring technologies may lead to more comprehensive and individualized healthcare experiences, improved health outcomes, and a more patient-centered approach to medicine [16].
Edge computing is critical in telemedicine and remote monitoring because it enables faster and more efficient data processing, which is especially significant when dealing with enormous volumes of health data supplied by various medical equipment.
According to a 2020 Dell survey, Healthcare and Life Sciences data increased about 900% in the previous two years, with 10 to 15 connected devices at the average hospital bedside [17]. Big data sets can quickly become overwhelming to efficiently manage, classify, and communicate. To be useful in the form of wearable devices and streamlined services, data must be processed and analyzed in real time, closer to the edge, and then sent to the cloud or an on-premises network when needed.
Edge computing is already having an impact since it allows healthcare practitioners to make faster, data-driven decisions and respond to patients’ needs in a timely manner. This is especially important in emergency situations and when monitoring patients with chronic illnesses because timely actions can make a major difference in health outcomes.
From pop-up clinics [18] and cancer screening centers [19] to patient-monitoring systems like pacemakers [20] and insulin pumps [21], there are hundreds of health-care applications that rely on low-latency, remote, real-time outcomes. While some technological applications are still in their infancy, edge computing will eventually help solve problems that cloud computing cannot.
The use of edge computing in telemedicine and remote monitoring is predicted to increase in the future as it enables the creation of more sophisticated medical devices and new healthcare solutions. It, for example, can offer real-time predictive analytics and AI-driven decision assistance, which can increase diagnosis accuracy and treatment effectiveness even further.
Furthermore, the combination of edge computing, 5G and upcoming 6G technology and the upcoming 6G has the potential to open up new avenues for remote surgeries, virtual reality-based medical training, and other game-changing healthcare applications. Overall, edge computing will continue to play an important role in improving telemedicine and remote monitoring capabilities, ultimately revolutionizing the way healthcare is given and experienced.
MedTech companies must transform amid disruption. Where do you begin?
The future of AI, ML, and DL in healthcare has enormous promise. But it is critical to understand present obstacles and constraints to provide a fair, secure, and effective healthcare system for all. To do that we need to ask ourselves: What are the challenges ahead and how to solve them to realize the full potential of these innovative technologies in healthcare?
We can work together toward a more equal and efficient healthcare landscape by embracing both the roses and the thorns in this field. Reach out to Milos Cigoj or one of our MedTech experts to learn how we can help you drive innovation of medical devices.
Stay tuned for the upcoming blog that will delve into the challenges and problems that go along with these extraordinary innovations and offer ways to overcome them.
In the meantime, learn more about Milos Cigoj’s outstanding experience in helping evangelize the importance of quality and compliance in medical device software development.
—
References
[1] Combining structured and unstructured data for predictive models: a deep learning approach – BMC Medical Informatics and Decision Making: 2020. https://bmcmedinformdecismak.biomedcentral.com/articles/10.1186/s12911-020-01297-6. Accessed: 2023-04-23.
[2] Artificial Intelligence (AI) in Healthcare Market Industry Report, Size, Growth Report, 2031: 2023. https://www.marketsandmarkets.com/Market-Reports/artificial-intelligence-healthcare-market-54679303.html. Accessed: 2023-04-23.
[3] Artificial intelligence in disease diagnosis: a systematic literature review, synthesizing framework and future research agenda: 2022. https://www.ncbi.nlm.nih.gov/pmc/articles/PMC8754556/. Accessed: 2023-04-23.
[4] Liu, X. et al. 2019. A comparison of deep learning performance against health-care professionals in detecting diseases from medical imaging: a systematic review and meta-analysis. The Lancet Digital Health. 1, 6 (Oct. 2019), e271–e297. DOI:https://doi.org/10.1016/s2589-7500(19)30123-2.
[5] Pai, R.K. et al. 2022. Quantitative Pathologic Analysis of Digitized Images of Colorectal Carcinoma Improves Prediction of Recurrence-Free Survival. Gastroenterology. 163, 6 (Dec. 2022), 1531-1546.e8. DOI:https://doi.org/10.1053/j.gastro.2022.08.025.
[6] Artificial Intelligence for Medical Diagnostics—Existing and Future AI Technology! 2023. https://www.mdpi.com/2075-4418/13/4/688. Accessed: 2023-04-23.
[8] Gould, M.K. et al. 2021. Machine Learning for Early Lung Cancer Identification Using Routine Clinical and Laboratory Data. American Journal of Respiratory and Critical Care Medicine. 204, 4 (Aug. 2021), 445–453. DOI:https://doi.org/10.1164/rccm.202007-2791oc.
[9] Nemanja, V. et al. 2022. BMC medicine 20 no. (2022).
[10] Artificial intelligence in drug discovery and development: 2020. https://www.ncbi.nlm.nih.gov/pmc/articles/PMC7577280/. Accessed: 2023-04-23.
[11] How AI Could Speed Drug Discovery | Morgan Stanley: 2022. https://www.morganstanley.com/ideas/ai-drug-discovery. Accessed: 2023-04-23.
[12] The Health ChatBots in Telemedicine: Intelligent Dialog System for Remote Support: 2022. https://www.hindawi.com/journals/jhe/2022/4876512/. Accessed: 2023-04-23.
[13] Telemedicine: Current Impact on the Future: 2020. https://www.ncbi.nlm.nih.gov/pmc/articles/PMC7502422/. Accessed: 2023-04-23.
[14] More Than Three-Quarters of Hospitals Anticipate Remote Patient Monitoring to Match or Surpass In-Patient Within Five Years: https://www.prnewswire.com/news-releases/more-than-three-quarters-of-hospitals-anticipate-remote-patient-monitoring-to-match-or-surpass-in-patient-within-five-years-301244169.html. Accessed: 2023-04-23.
[15] The Rise of Consumer Health Wearables: Promises and Barriers: 2016. https://www.ncbi.nlm.nih.gov/pmc/articles/PMC4737495/. Accessed: 2023-04-23.
[16] The Impact of Wearable Technologies in Health Research: Scoping Review: 2022. https://mhealth.jmir.org/2022/1/e34384. Accessed: 2023-04-23.
[17] Edge and IoT solutions: https://www.delltechnologies.com/asset/en-us/solutions/industry-solutions/briefs-summaries/dell-technologies-edge-iot-healthcare-infographic.pdf. Accessed: 2023-04-16.
[18] Edge computing delivers healthcare beyond the clinic: http://www.ft.com/partnercontent/ntt-ltd/edge-computing-delivers-healthcare-beyond-the-clinic.html. Accessed: 2023-04-24.
[19] Edge detection algorithm of cancer image based on deep learning – PubMed: 2020. https://pubmed.ncbi.nlm.nih.gov/32564648/. Accessed: 2023-04-24.
[20] AI cardiologist at the edge: A use case of a dew computing heart monitoring solution: 2022. https://www.sciencedirect.com/science/article/abs/pii/B9780128240540000204?via%3Dihub. Accessed: 2023-04-24.
[21] Insulin dose optimization using an automated artificial intelligence-based decision support system in youths with type 1 diabetes – Nature Medicine: 2020. https://www.nature.com/articles/s41591-020-1045-7/. Accessed: 2023-04-24.