Use of digital twins is becoming increasingly prominent in the design and operation of cyber-physical systems. This blog briefly describes the concept and use of digital twins in Internet of Things (IoT) industrial systems, their origins, current trends, and futures projection.
The term digital twin in IoT generally refers to a synchronized software representation and replica of a physical object or process (physical twin). A digital twin has a real-time connection to sensors and data from its physical counterpart that allows it to continuously mirror its states and behaviors in the virtual representation.
The concept and the term digital twin were originally used by NASA to describe the hybrid replicas of their vehicles that combined physical artifacts, precise multi-physics models, and live sensor data to mimic its physical twin in space. The earthly twin was used to simulate, analyze, and predict behaviors of the physical counterpart operating in space in order to plan control actions, diagnose and mitigate problems.
The origin
Early published implementations of IoT digital twins, such as in AWS (called device shadow) and Azure IoT platforms, focus primarily on cloud representation of basic endpoint things. In those incarnations, an IoT digital twin is basically a data structure in the cloud that represents physical things, such as sensors and devices. Twins receive data and status updates from their thing counterparts and thus mirror the thing states as close to real time as possible.
IoT digital twins often have representations of the actual as well as of the desired states of the device. Applications and services may modify the desired state to cause the twin implementation to issue the necessary commands to the device to modify its state accordingly and to bring the two into compliance.
Cloud-based applications and services can use and access digital twins as device proxies instead of accessing the endpoints directly. This mode of operation has several potential advantages, including: 1) faster access, (2) continuous availability, (3) savings of bandwidth and power, (4) an abstract representation and interface.
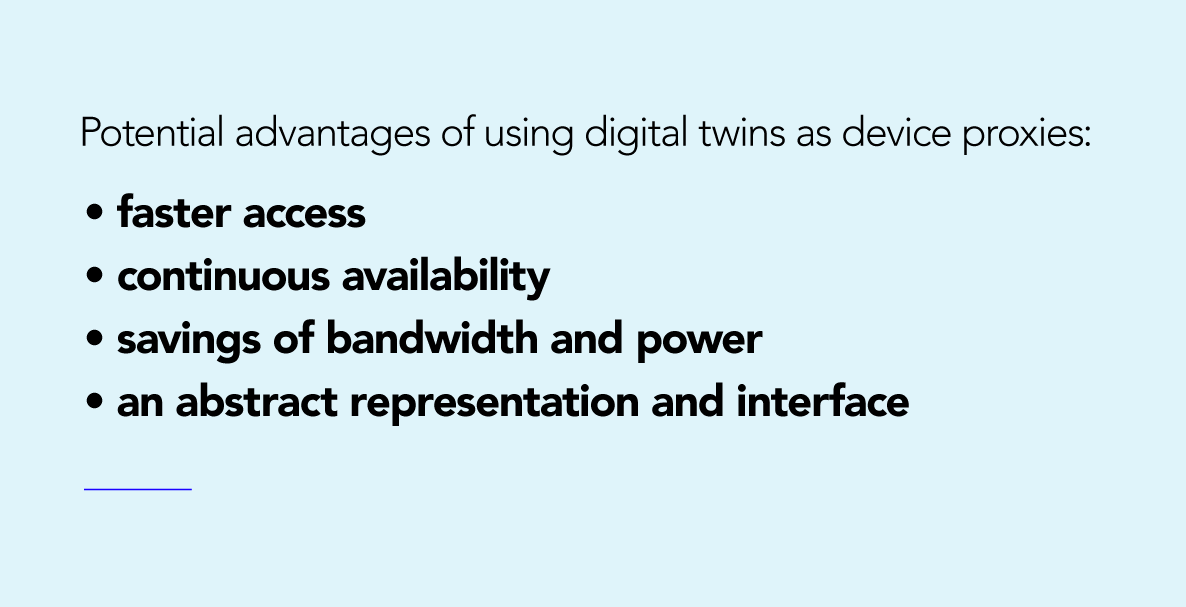
Cloud-hosted digital twin can be accessed by the cloud applications on demand and without the latencies involved in accessing the actual device or waiting for it to report. Obviously, this access obtains the last reported thing state which is not necessarily the current one. That might be OK in many cases since instant synchrony in distributed systems is not possible anyway due to the inevitable communication delays.
The digital twin state representation is always available, even during periods of time when the physical device is disconnected or sleeping. This allows delay-tolerant applications to proceed without having to wait for the temporarily inaccessible devices.
Savings of bandwidth at the edge and on the way to the cloud occur when the access to digital twins can substitute for direct access to the devices. Bandwidth is usually in ample supply in the cloud where the digital twins reside, but it may be costly or restricted at the edge. Power may be saved in constrained environments by not forcing the device to wake up and to power up its transceiver to send a report.
Abstract representation refers to the uniform data and command formats that digital twins can maintain for cloud applications to query thing data or to issue instructions to change its desired state. Such an approach can simplify implementation and increase portability of applications by hiding device differences, intricacies and details from the cloud applications. This is accomplished by using the twin implementations to translate between the generic representations and the device-specific data formats and commands.
This type of usage of digital twins is now commonplace in IoT systems and it remains useful primarily for monitoring applications with some limited simulation and prediction capability when enhanced by models and AI.
Now(ish)
Having proven their usefulness in practice, development of digital twins in IoT is now expanding to incorporate elaborate behavior models by using true-to-reality physics and even 3D photorealistic rendering.
Addition of physically accurate behavioral models makes digital twins the exact virtual replicas of their real-world twin counterparts. They maintain state synchronicity with the physical twin via real-time bidirectional communication. This enables digital twins to be used for living simulations with precise timing. They in turn allow for elaborate “what if” types of analyses to obtain additional insights and predict outcomes of changes as well as cope with disturbances such as malfunctions, failures, and drastic changes in the operating environment.
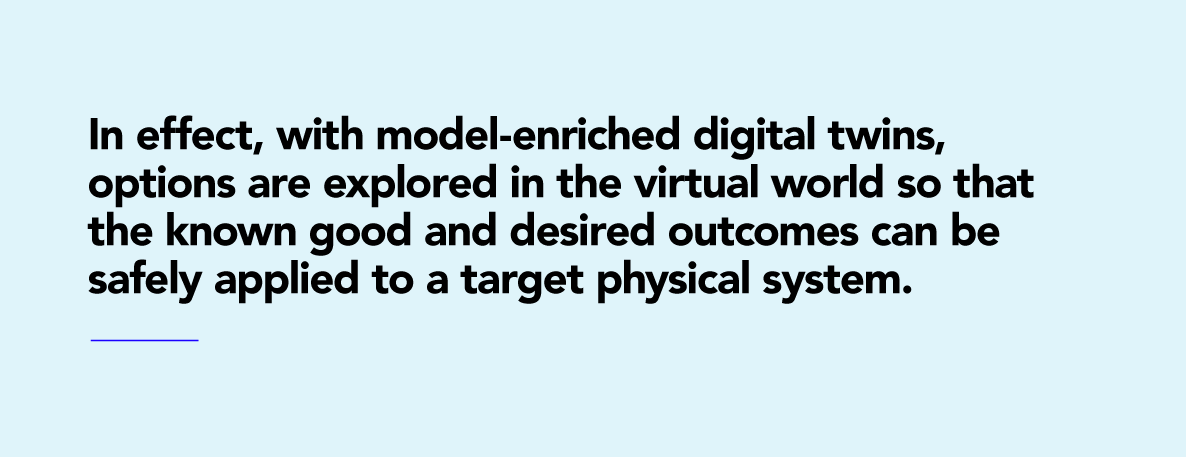
System simulations can be fed with real-time data or with historical traces of the system under observation. The latter is useful for testing insights and assessing potential value of optimizations on the past system behaviors, as well as for the root cause analyses of past failures so that they can be identified and rectified. In effect, with model-enriched digital twins, options are explored in the virtual world so that the known good and desired outcomes can be safely applied to a target physical system.
Models used in digital twins are personalized in the sense that they accurately represent a specific physical counterpart rather than just a broad category. Owing to constant synchrony and real-time connection, digital twins can make use of data assimilation to evolve and accurately reflect changes in their digital twin, such as ageing. A piece of machinery can change its properties after being in use for a period of time, and its digital twin can model those to make predictions suited to its actual state. This concept can be expanded to many uses, such as creating data-driven evolving digital twins of humans for personalized healthcare.
Digital twins can also be enhanced with various levels of rendering, all the way up to 3D photorealistic rendering. An example of a collaborative robot with rendering attached to its digital twin is provided in the figure below (image courtesy of NVIDIA).
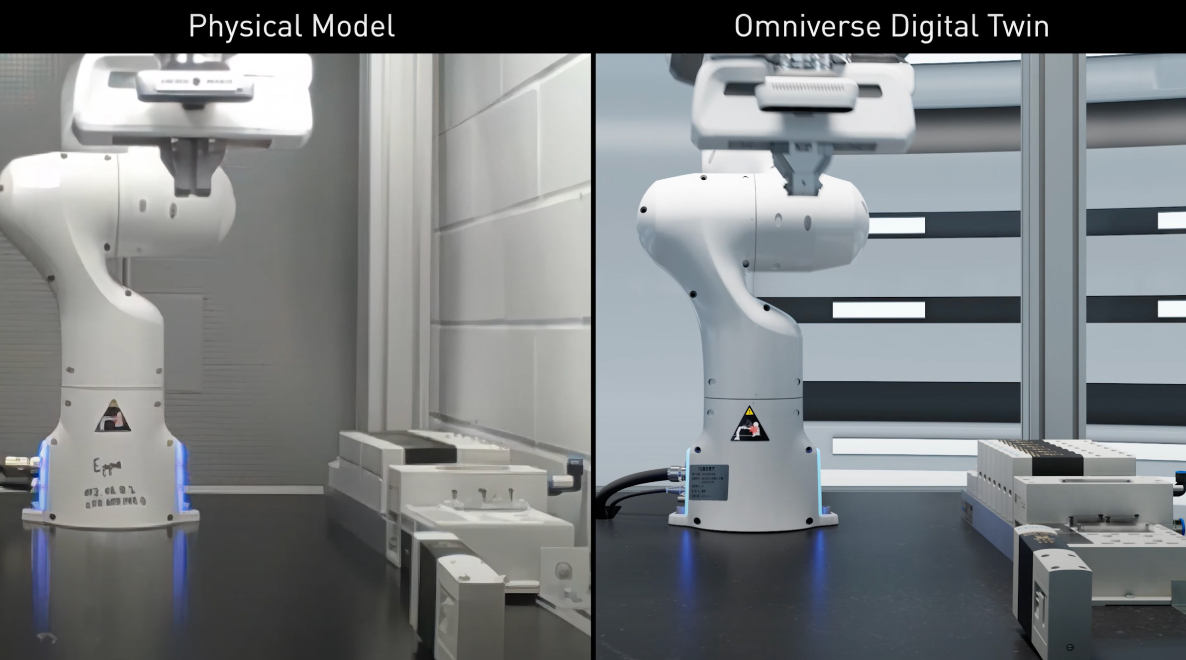
(source: NVIDIA)
Rendering in digital twins is useful for visualization and local and remote viewing which is convenient for humans and can lead to additional insights. For example, it can be used for exploring the effects of various motions in robots and applying the safe and effective ones to their training.
Digital twins with accurate physical models and rendering form the elements of the virtual experience that is often referred to as the industrial metaverse. This is somewhat similar in appearance to the social network metaverse. The key difference is that social metaverses exist primarily in the virtual world with actions mostly driven by human users, whereas the industrial metaverse contains virtual elements that are exact replicas of real-world entities designed to continuously mimic their behaviors in a synchronized manner. The term omniverse is sometimes used to refer to the two synchronized physical and virtual realities.
Practical considerations
As described, digital twins can be very useful in industrial applications with automated manufacturing lines that tend to be closed-loop systems with largely deterministic behaviors that can be modeled. Some of the proponents of digital twins project the application of this concept to large complex systems that may involve people, social interactions, and the natural environment. All of these expectations should be tempered with practical considerations.
Modeling can be costly, especially for complex components and systems. This is especially the case when no design CAE or CAD models are available, and it must be done from scratch. Complex, multi-component or spatially expansive systems, such as manufacturing lines or warehouses may require the use of laser scanning to create just the surface-level model. The extent of modeling and scope of digital twins in practical applications must be guided by the measurable benefits and return on investment. Detailed rendering can be demanding to develop, so it should be done where necessary, such as in visualizing and planning robot movements.
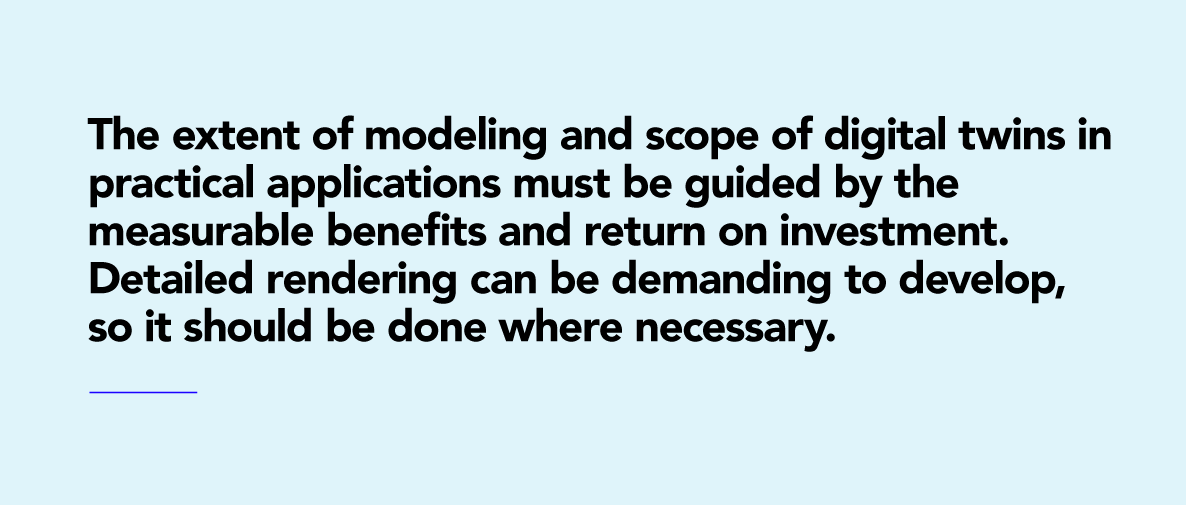
As the statistician George Box pointed out, “… all models are wrong, but some are useful”. Even when the underlying physics is well understood, models are and should be treated as approximations with various degrees of fidelity affected by balancing accuracy with computational complexity. They are driven by data that are often incomplete due to partial physical coverage of sensors, inferred rather than direct measurements, measurement errors, and data loss. Thus, in practice, models are rarely a completely accurate representation of the physical world, and their predictions should be treated with a degree of uncertainty, preferably quantified. The uncertainty grows considerably when human behaviors, such as the workers on the manufacturing line, must be taken into consideration.
And beyond
Beyond the operational use described thus far, digital twins can add value in all stages of a product life cycle. A manufacturer can use accurate physical models and simulation to design and optimize a product in the virtual domain before settling on the final version for production. Finalized models can then be used to create digital twins for operational purposes by connecting them to the data from the related equipment as it is installed.
Once deployed, the data and insights from the digital twin can be used not only by the system operator but also by the equipment manufacturer to track real-world behaviors of their products over large swaths of the installed base. For example, an aircraft engine manufacturer can monitor and track their fleet in operation. This is beneficial to both the manufacturer and its customers. Operators, such as airlines, can benefit in terms of receiving expert guidance on preventive maintenance and handling of emergencies.
In turn, the manufacturer can use the data from the actual operating conditions in the real world to refine their ML and AI algorithms on large sets of actual data and use those to improve future generations of the product. These kinds of arrangements also enable creation of new business models, such as power as a service.
Completing the product life cycle, digital twins can also assist in the decommissioning process when the equipment is retired or repurposed. By traversing different stages of product lifecycle, digital twins can provide a way to bridge a long-standing gap between design, commissioning, and usage phases of equipment by sharing the same model and thus communicating the design intent to system operators.
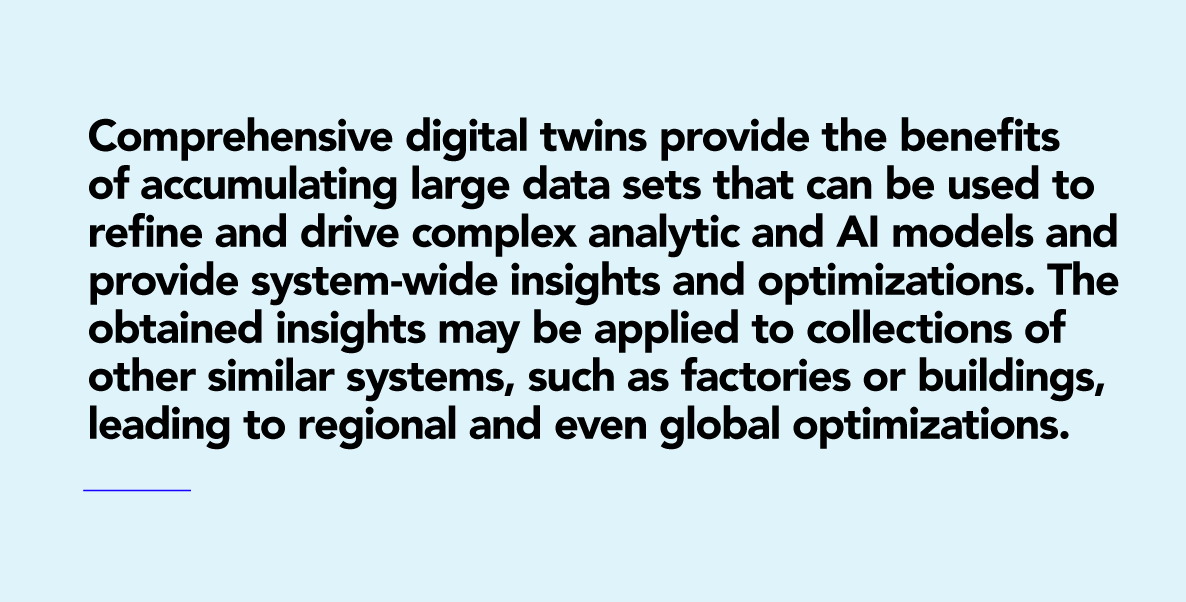
Synchronized and physically accurate digital twins are shaping up to be a powerful tool for monitoring and safely and efficiently managing a wide range of devices and processes. While the IoT system builders are busy trying to create and make them work, the current excitement is about expansion from the individual devices to compositions, ranging from groups of connected objects such as machinery on the production floor, to complex systems such as factories, buildings and ships. These are usually compositions of individual digital twins for component parts, such as machinery and robots. The aggregate digital twin also replicates the corresponding component interconnections in the physical system to accurately reflect the entirety of its data and control flows – basically echo the defining ensemble behaviors.
Such comprehensive digital twins provide the benefits of accumulating large data sets that can be used to refine and drive complex analytic and AI models and provide system-wide insights and optimizations. Furthermore, the obtained insights may be applied to collections of other similar systems, such as factories or buildings, leading to regional and even global optimizations while drawing on even larger data sets for further improvements.
Some proponents of this idea advocate creating digital twins of complex systems beyond the manufacturing domain, e.g., for traffic, cities, healthcare, and even parts of the natural systems that surround us. The hope is to improve many aspects of human activities including understanding of the natural world we live in. Such digital twins may have to learn models by observation and data assimilation and include uncertainties such as human behavior which can be hard and unpredictable.
Experience with the industrial IoT uses shows the great potential and benefits of model-enhanced digital twins, the tools for creating them are being developed, leaving it to imagination and experimentation to find out how broadly and to what purposes they can be usefully applied.
You can read more of Milan’s observations on the Internet of Things on his blog, iotsense.com.