Artificial intelligence (AI), machine learning (ML), and deep learning (DL) have enormous potential to alter the healthcare sector, bringing significant advancements in diagnostics, tailored treatment, and efficient patient care. To ensure the responsible and ethical development and application of these technologies, it is crucial to acknowledge the challenges and problems that go along with these extraordinary innovations.
Following the recent article about Top trends shaping the medical device industry, today we will look at the numerous obstacles that AI, ML, and DL face in the healthcare domain, such as data protection, ethical implications, and potential biases, as well as regulatory issues, workforce adaptation, and liability concerns.
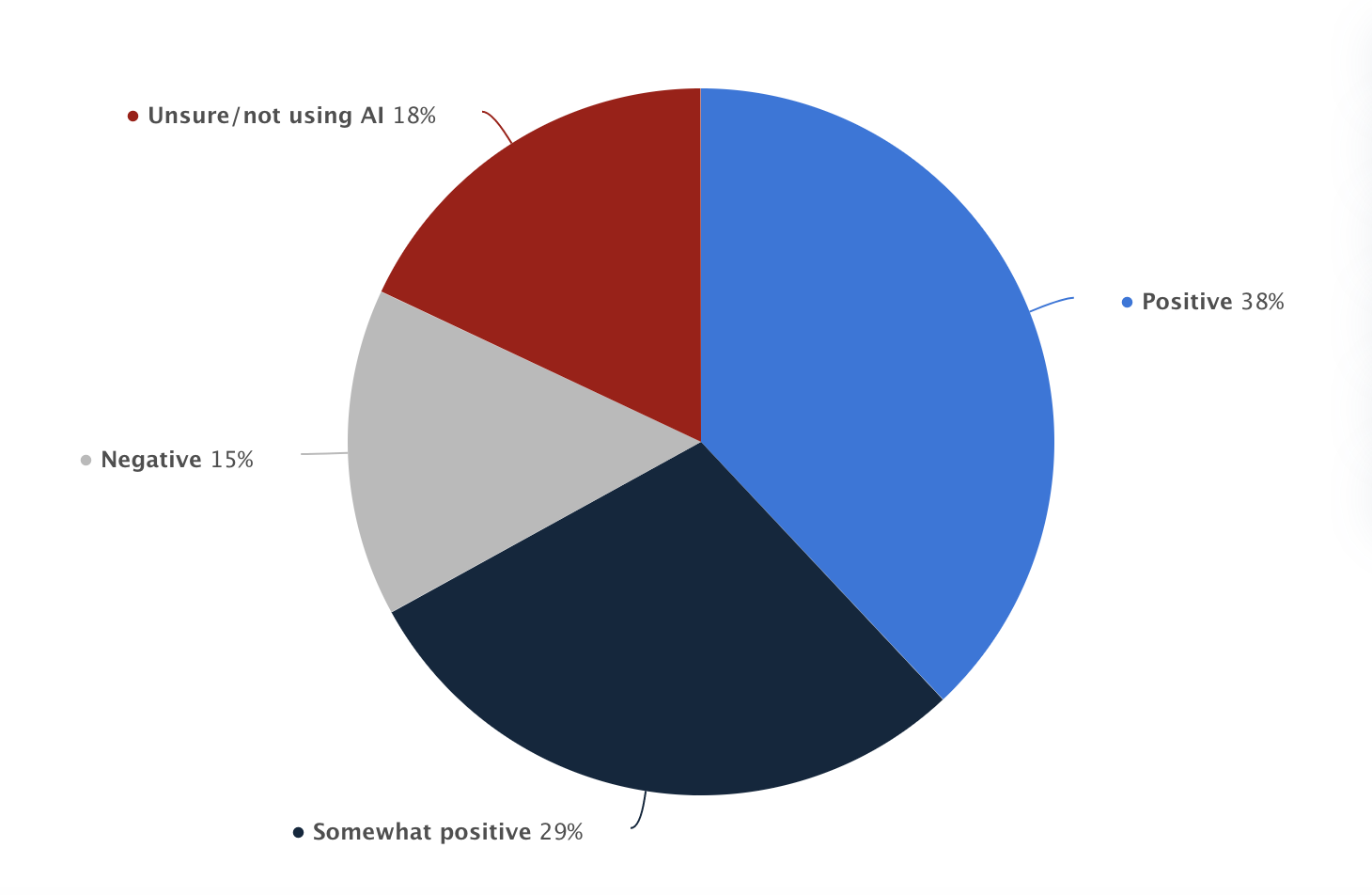
Graph representing the perception of regulations for AI in health care among U.S. health leaders as of 2020 by Statista
The growing importance of cybersecurity in connected devices
The growing importance of cybersecurity for connected devices, especially in the healthcare sector, can be attributed to the increasing reliance on digital technologies and the widespread adoption of Internet of Things (IoT) devices. As more medical devices become interconnected, they become more vulnerable to cyber threats. The evolution of cybersecurity on connected devices will involve several key aspects:
Regulatory compliance
Pursuing regulatory compliance with purpose is imperative for medical device companies. Governments and regulatory bodies, such as the FDA [1] and the European Union [3], have recognized the need for cybersecurity in medical devices and have issued guidelines [2] and requirements for manufacturers. In the future, we can expect more stringent regulations and mandatory compliance, which will drive medical device manufacturers to prioritize cybersecurity in their products. Cyber-attacks are recognized as a hazardous situation that can lead to patient harm. This is why total product lifecycle management must include risk management that assesses cybersecurity aspects [2].
Risk assessment and management
Risks related to cybersecurity threats and vulnerabilities should be evaluated at all stages of a medical device’s life cycle, from the conception to end-of-support. Risk management should be implemented across the total product life cycle (TPLC), where cybersecurity risk is analyzed and mitigated throughout the design, manufacturing, testing, and post-market monitoring activities, to effectively manage the dynamic nature of cybersecurity risk.
A cybersecurity risk that jeopardizes device safety and essential performance, impairs clinical operations, or causes diagnostic or therapeutic errors should also be evaluated in the risk management process for a medical device innovation. This is reflected in AAMI TIR57:2016 [4], which suggests that the risks associated with a device’s cybersecurity include harm to patient safety (as defined in ISO 14971) and can be associated with indirect patient harm via cybersecurity security risks.
Encryption and secure communication
As the healthcare industry increasingly relies on digital technology and interconnected devices, it is critical to use strong encryption and secure communication protocols and standards to prevent cybersecurity concerns. To protect sensitive patient data both in transit and at rest, the industry must focus on implementing advanced encryption approaches such as homomorphic encryption and quantum-resistant algorithms in the future. Furthermore, the significance of HL7 (Health Level Seven International) and IHE (Integrating the Healthcare Enterprise) guidelines implementation in the healthcare sector cannot be emphasized. These standards aid in the seamless and secure sharing of patient data between various healthcare information systems, increasing interoperability and improving overall healthcare delivery efficiency.
HL7 is a set of international standards for the exchange of clinical and administrative data between different types of healthcare IT systems. Using HL7 standards ensures that diverse systems interact successfully, lowering the chance of data loss or misinterpretation during transmission. This is crucial as the healthcare industry continues to digitalize and use electronic health records (EHRs).
IHE, on the other hand, is a project focused on enhancing the way computer systems in healthcare exchange data. It establishes a framework for the coordinated application of known standards, such as HL7 and DICOM (Digital Imaging and Communications in Medicine), to address specific clinical data sharing needs. Implementing IHE principles ensures that the healthcare sector takes a consistent approach to integrating disparate systems, encouraging collaboration, and improving patient care.
Healthcare businesses can improve the security and efficiency of their data interchange operations while also supporting better decision-making and patient outcomes by following HL7 and IHE principles. These principles also contribute to the development of a more robust healthcare ecosystem in which all stakeholders may securely and effectively access and use patient data, eventually leading to enhanced patient care and safety.
Standardized security frameworks, such as the NIST Cybersecurity Framework and the ISO/IEC 27001 standard, can also help firms manage and reduce cybersecurity risks.
Authentication and access control
As medical devices become more interconnected, it is crucial to implement strong authentication and access control mechanisms to prevent unauthorized access. This may involve using multi-factor authentication, unique device identifiers, and role-based access controls to ensure that only authorized personnel can access and control the devices.
Security updates and device management
Medical device manufacturers will need to establish processes for providing timely security updates and patches to address newly discovered vulnerabilities. This will involve monitoring security threats, developing and testing patches, and ensuring that devices can be updated remotely and with minimal disruption to healthcare services.
The following graph represents the number of healthcare data breaches involving the loss of 500 or more records in the United States from 2009 to 2021.
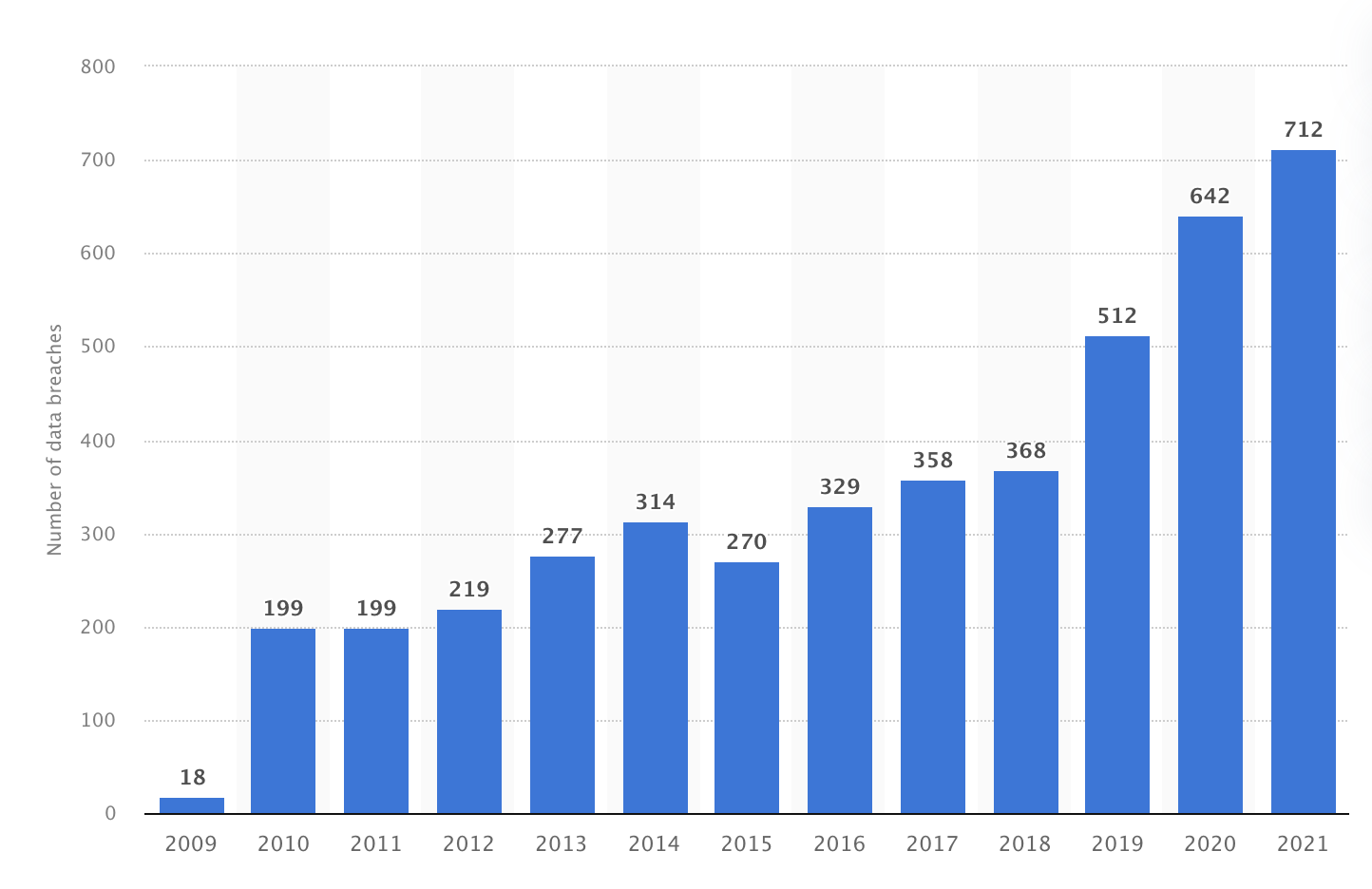
Source: Statista
Explainable AI
The phrases explainability and interpretability are frequently used interchangeably in science, yet they have significant differences. Although explainability and interpretability don’t have a formal mathematical definition, there have been several attempts to distinguish between the two ideas. The most frequently used definition of explainability is the capacity to communicate with people in understandable terms. On the other hand, the capacity to understand the concepts underlying a model’s outputs directly affects how interpretable its results are.
Explainable AI (XAI) makes it easier for users to understand and accept computerized decisions [26]. A more understandable model might aid in improving our knowledge of human disorders.
The internal logic or underlying mechanics of an interpretable model may be incomprehensible to humans. As a result, interpretability does not necessarily imply explainability in the context of machine learning systems, and vice versa.
As a result, it has been suggested that explainability is also necessary and that interpretability alone is insufficient. To fully comprehend XAI, a range of models are provided. For instance, interpretable and interactive machine learning modeling techniques that simultaneously incorporate machine learning professionals and domain experts have been used in healthcare systems.
The following list summarizes the benefits of opening a window into these black-box systems [5]:
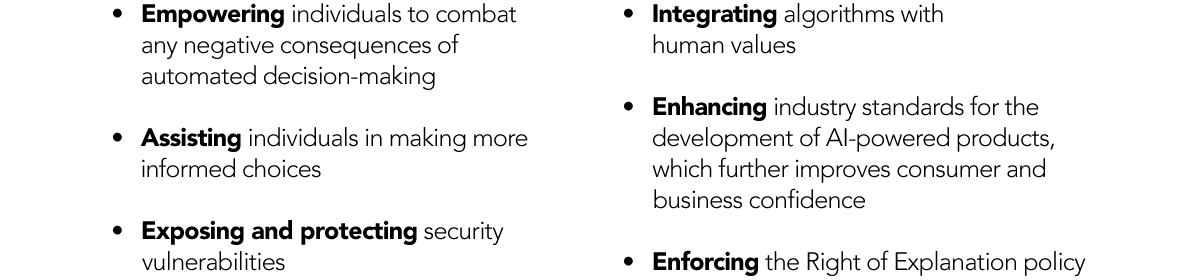
Lack of curated data
To be effective, AI systems need a vast amount of high-quality, tagged data. The medical industry may experience problems as a result since data is usually incomplete, fragmented, unlabeled, or unavailable. Due to the poor quality and dearth of medical data, particularly in AI applications, it poses a significant challenge for all stakeholders. To assure the accuracy and efficacy of AI-driven technologies, which have the potential to change healthcare by assisting in diagnoses, treatment planning, and medication discovery, trustworthy training data is critical. Obtaining high-quality, annotated data, on the other hand, is hampered by a variety of variables, including patient privacy concerns, data fragmentation across various healthcare systems, and the lack of standardized formats.
Biased AI
Poor data is one evil, but there is more. AI models may be trained on outdated, or incomplete datasets, undermining their effectiveness and hindering the advancement of AI-driven medical solutions. On top of this if AI algorithms are trained on data that is not representative of the people they are intended to serve, they may be biased. Using algorithms to make medical decisions can result in an unfair or inaccurate diagnosis.
Bias is not a new problem; it can be compared to a one-way mirror in which a population only sees its own perspective and is unaware of the views of other groups. In this comparison, the population’s point of view is mirrored back at them, supporting their own opinions and perspectives while other groups’ points of view remain invisible or concealed. This one-way mirror effect leads to a limited knowledge of the world, propagating prejudices and ignoring other people’s unique experiences and needs. Bias in human nature and AI systems can impede fair decision-making and equitable treatment of diverse groups in the same way that a one-way mirror presents a barrier to true comprehension and empathy.
AI-based decision-making, on the other hand, has the potential to reinforce existing prejudices while also altering new categories and situations, potentially resulting in new types of bias. As a result of these developing concerns, AI-based algorithms are being re-evaluated to implement new methods that address the objectivity of their judgments. The most recent technologies for bias in AI-based decision-making systems are investigated, as are open issues and guidelines for public-good AI solutions.
Bias has to be addressed in the various stages of AI decision-making by pre-processing, processing, and post-processing strategies, with pre-processing, processing, and post-processing methods concentrating on data entry, learning algorithms, and model results, respectively.
Since bias and discrimination can be diverse and flexible, these issues necessitate multidisciplinary approaches and continual interaction with society. However, as AI technology becomes more prevalent in our lives, it is critical for technology makers to recognize bias and prejudice and ensure responsible technology use, keeping in mind that technology alone is not a solution to all forms of bias and AI concerns.
Ethical implications
The application of generic artificial intelligence in medical diagnostics on a private and sensitive dataset raises a number of ethical concerns in terms of data privacy, algorithmic transparency, and responsibility for making AI-algorithmic conclusions. Before its capabilities may be used in medical research, the device must be put through additional testing [6].
The effects of ethical behavior on the existence and usage of digital devices are the focus of technology ethics. AI has a wide range of behavioral consequences in healthcare.
The first behavioral issue concerns AI’s ethical responsibilities. Accepting responsibility for one’s conduct is a moral imperative. Some may argue that because AI is sensitive, they have no moral obligation. However, it is vital to recognize that AI may be morally accountable. The computer software employed in medical examinations, for example, is not emotional, but it has a moral obligation to be.
The second moral blunder is the AI developer’s fault. It is in charge of ensuring that AI can meet people’s demands.
The third behavioral barrier is the responsibility that comes with utilizing AI. It is our obligation to ensure that artificial intelligence is not utilized for unethical ends.
The fourth behavioral issue is responsibility for humans harmed by AI. AI is in charge of ensuring that it does not have a negative impact on a specific set of individuals or society.
The fifth definition of ethics is the responsibility that comes with using artificial intelligence. It is our responsibility to ensure that artificial intelligence is not utilized in ways that violate the rights of others. The sixth ethical conundrum is accountability [28], which is connected with the ethics used to govern AI design.
These concepts are used to assist AI developers in ensuring that their AI works according to ethical concerns.
Professional liability
Historically, credentialed medical specialists in a particular specialty have been in charge of making clinical choices. Because AI is frequently employed to aid with clinical operations, AI decision support systems may have an impact on healthcare practitioners’ professional obligations with regard to each individual patient. The legal duty of AI-assisted decisions is generally misunderstood, which is problematic given that AI is capable of forming incorrect conclusions. The fact that developing pertinent legal concepts and rules takes a much longer time than developing technological skills makes this situation worse. Another issue is that AI will deter medical personnel from undertaking quality assurance tests and may make it difficult for them to identify faults [8].
A damage induced by a physician’s divergence from the standard of care leads to medical malpractice. The combined actions of the physician’s professional peers, depending on local or national criteria, determine this standard of care.
A physician who relies on an AI system in good faith to offer suggestions may nonetheless face liability if the practitioner’s actions fall below the standard of care and other criteria of medical malpractice are met. Regardless of the result of an AI algorithm, physicians have an obligation to independently apply the standard of care for their profession. While case law regarding physician use of AI is still in its early stages, multiple lines of cases show that physicians share the responsibility for errors caused by AI output [9].
AI is now employed as a decision support tool by healthcare professionals, allowing them to make better educated and correct decisions while maintaining full professional accountability. However, the dynamics of accountability may shift as the legislative system evolves and catches up with rapid technological advancements. It is critical to monitor and react to these advances, ensuring that both healthcare professionals and AI systems collaborate to offer the best possible patient care while navigating the evolving landscape of liability and legislation.
Closing remarks
We examined and highlighted the problems and barriers to mainstream AI use in healthcare, such as cybersecurity, data privacy, AI explainability, and bias. The necessity of resolving ethical issues about data privacy, algorithmic transparency, and accountability was underlined, as was the need for collaboration among healthcare providers, manufacturers, and regulatory agencies.
In conclusion, the future of AI, ML, and DL in healthcare has enormous promise, but it is critical to overcome present obstacles and constraints to provide a fair, secure, and effective healthcare system for all. Continuous development and refining of AI-based medical diagnostic systems, acceptance of interoperability protocols, and consideration of ethical issues are crucial to realizing the full potential of AI technology in healthcare.
We can work together to construct a more resilient, inclusive, and ethically sound healthcare system by recognizing and addressing these difficulties head-on and harnessing the power of AI, ML, and DL to enhance patient outcomes and alter the way we approach medicine. We can work toward a more equal and efficient healthcare landscape by embracing both the roses and the thorns in this field.
—